AI is making financial analysis faster and smarter. It helps with things like analysing data, making better predictions, and spotting trends that might go unnoticed.
In this blog, we’ll show you 10 AI tools to transform financial analysis that can help traders, investors, and financial experts get sharper ideas and improve their decision-making.
The 10 Best AI Tools for Smarter Financial Analysis
Here are 10 AI tools to transform your financial analysis:
1. Datarobot
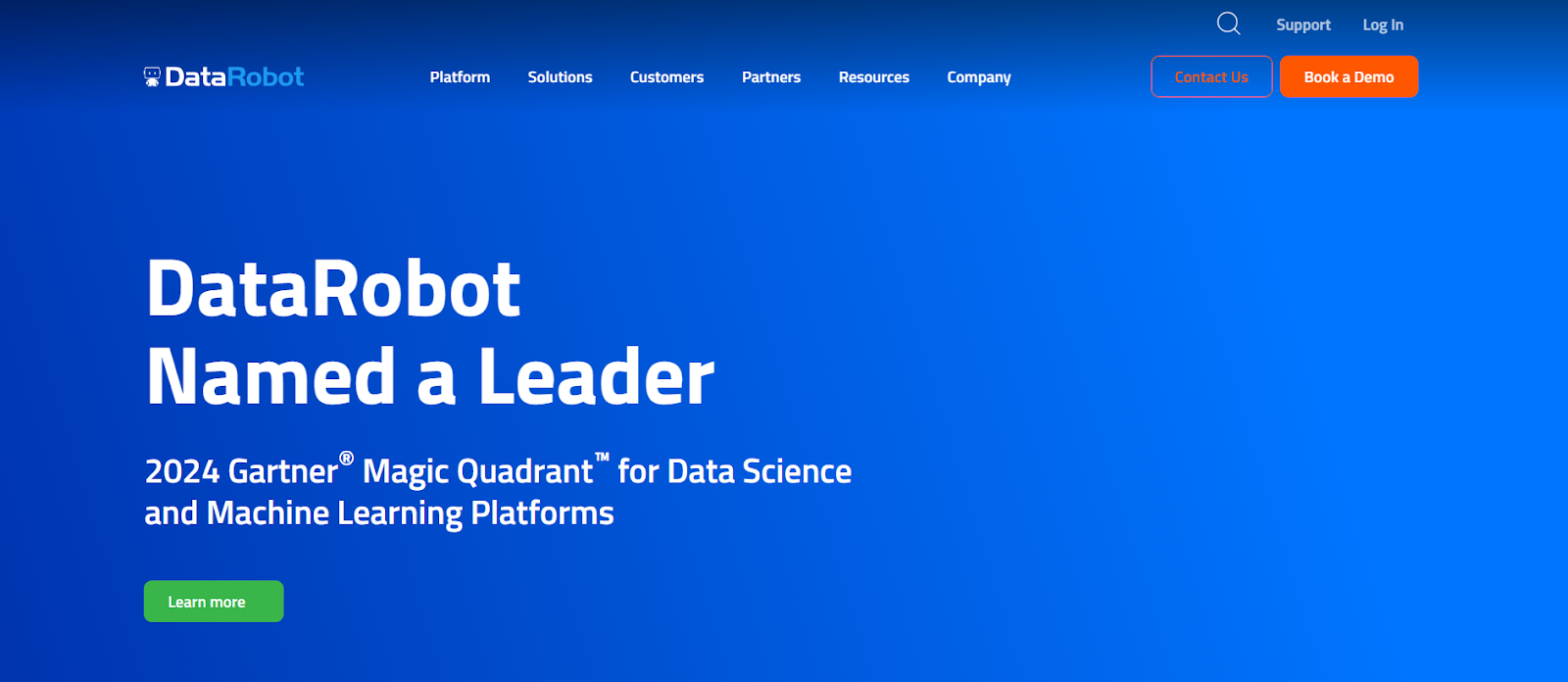
Datarobot is an AI-powered platform that automates the process of building and deploying predictive models. Can be used for a variety of financial analysis tasks. Including fraud detection Risk assessment and notifying customers
Key Points:
- Automated Machine Learning: Building Models with Extensive Data
- Knowledge Timeline Forecasts: Predictions of future financial trends and returns.
- Identification of Anomalies: Identify unusual patterns, trends or outliers in financial data.
- Linking to various resources: Connect to various resources. for analysis
Pros:
- Its Users usually work with the minimum code required.
- There are many machine learning algorithms out there.
- It Can support large data sets and complex models.
- It can mix with other media very well.
Cons:
- It may require preparation and cleaning before use.
- It can be expensive for large organisations
- Its highly customised models may have limitations.
- It may not be appropriate for companies with highly structured data.
More Info: DataRobot
2. Alteryx
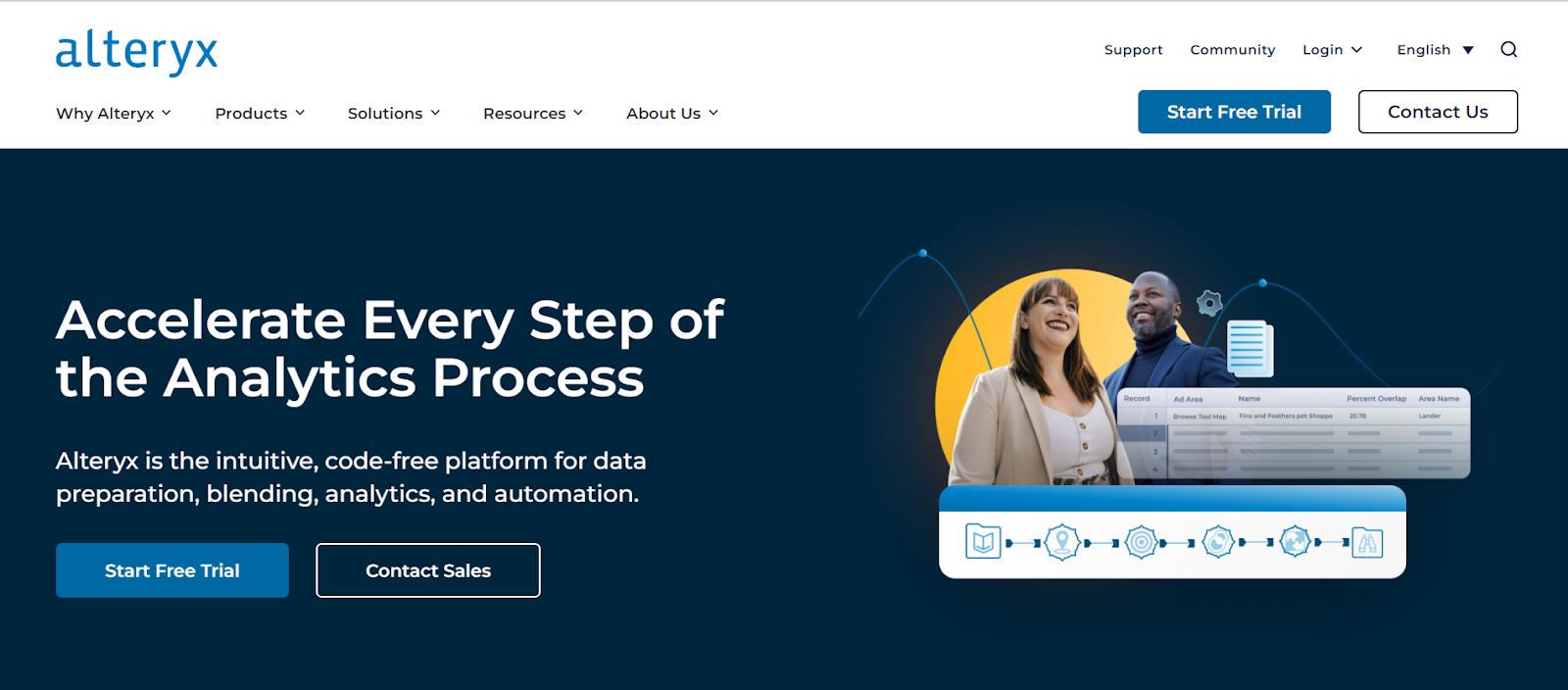
Alteryx is a data analytics platform that provides a drag-and-drop interface for creating and automating workflows. It can be used for various financial analysis tasks, such as financial reporting, budgeting and predictions
Key Points:
- Create workflows without writing code.
- Combine and clean data from different sources.
- Create predictive models for financial forecasting.
- Connect different data sources for analysis.
Pros:
- Easy to learn and use even for beginners.
- There are a variety of data analysis tools available.
- Its able to manage large data sets and complex workflows.
- Can integrate with other data science tools very well.
Cons:
- They can be much less flexible than code-based tools.
- It can be expensive for large organisations
- Highly customised workflows can have limitations
- May not be suitable for industries with highly regulated data
More Info: Alteryx
3. RapidMiner
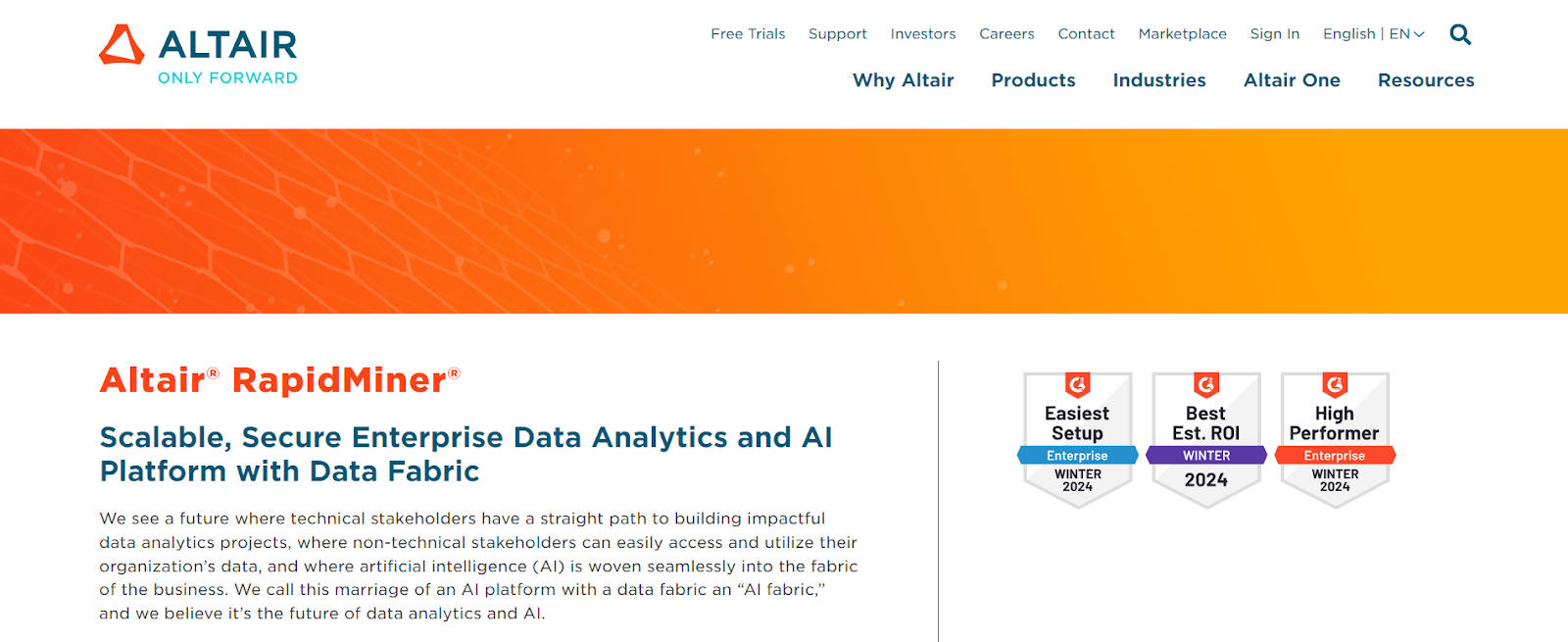
RapidMiner is a data mining platform that provides a variety of tools for mining data, machine learning and predictive analytics . It can be used for a variety of financial analysis tasks. Including fraud detection, risk assessment and notifying customers
Key Points:
- It creates models without writing code.
- Activities such as data cleaning.
- It achieves additional algorithms for modelling.
- Connect to various resources. for analysis
Pros:
- This tool can be used in several ways.
- Able to provide a variety of information services
- It has both drawing and writing features.
- It mixes with other media very well
Cons:
- There may be a steeper learning curve compared to other tools.
- May be more expensive for commercial use
- Highly customised models may have limitations.
- May not be suitable for industries with highly regulated data
More Info: Data Analytics and AI Platform | Altair RapidMiner
4. KNIME Analytics Platform
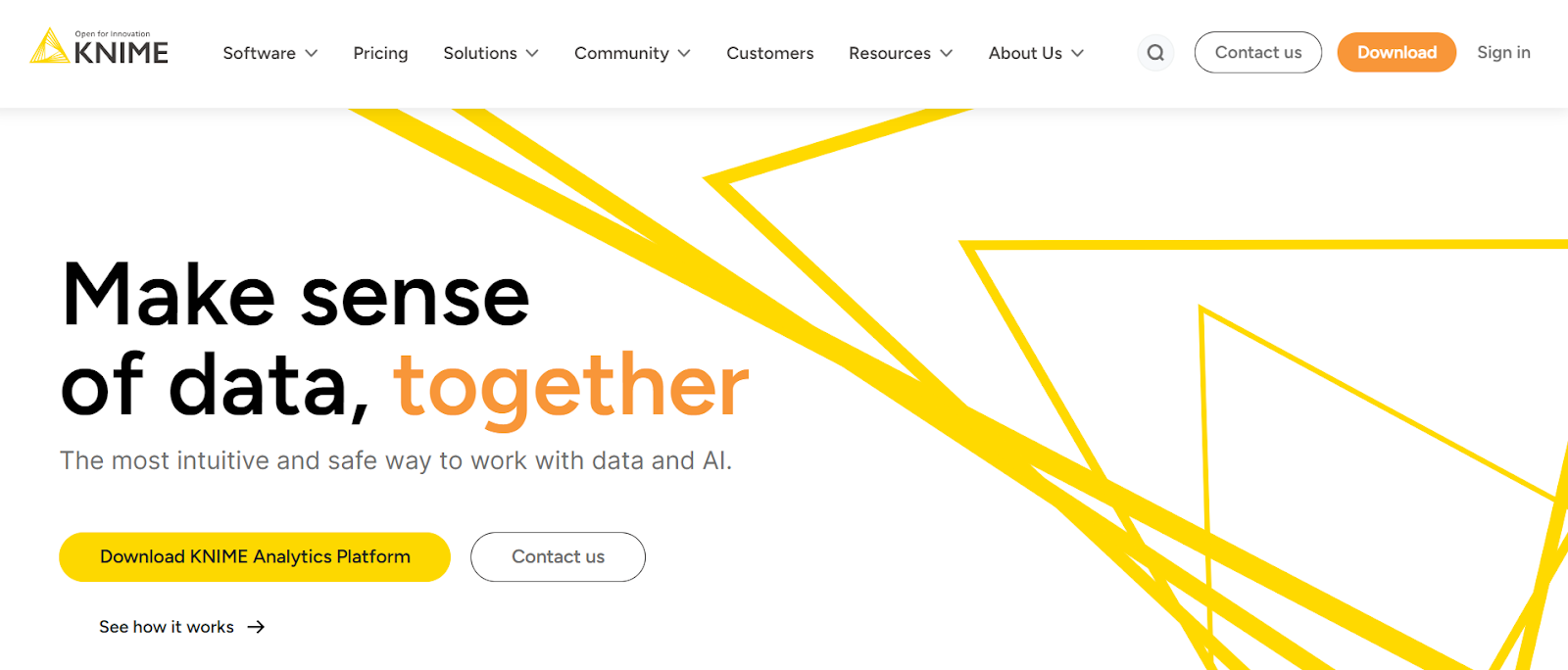
KNIME is an open source database that provides a functional computational platform for building and deploying predictive models. It can be used for a variety of financial analysis tasks. Including fraud detection and notifying customers.
Key Points:
- free and customisable
- Perform data cleaning.
- Access to algorithm libraries for modelling.
Pros:
- Very flexible and adjustable
- It can be used in many data science applications.
- A great and enthusiastic community for support and resources.
- It integrates well with other open source tools.
Cons:
- It can be a steep learning curve for beginners.
- Additional technical expertise may be required for complex applications.
- There may be limitations for highly customised models.
- May not be appropriate for companies with highly structured data.
More Info: KNIME
5. Orange
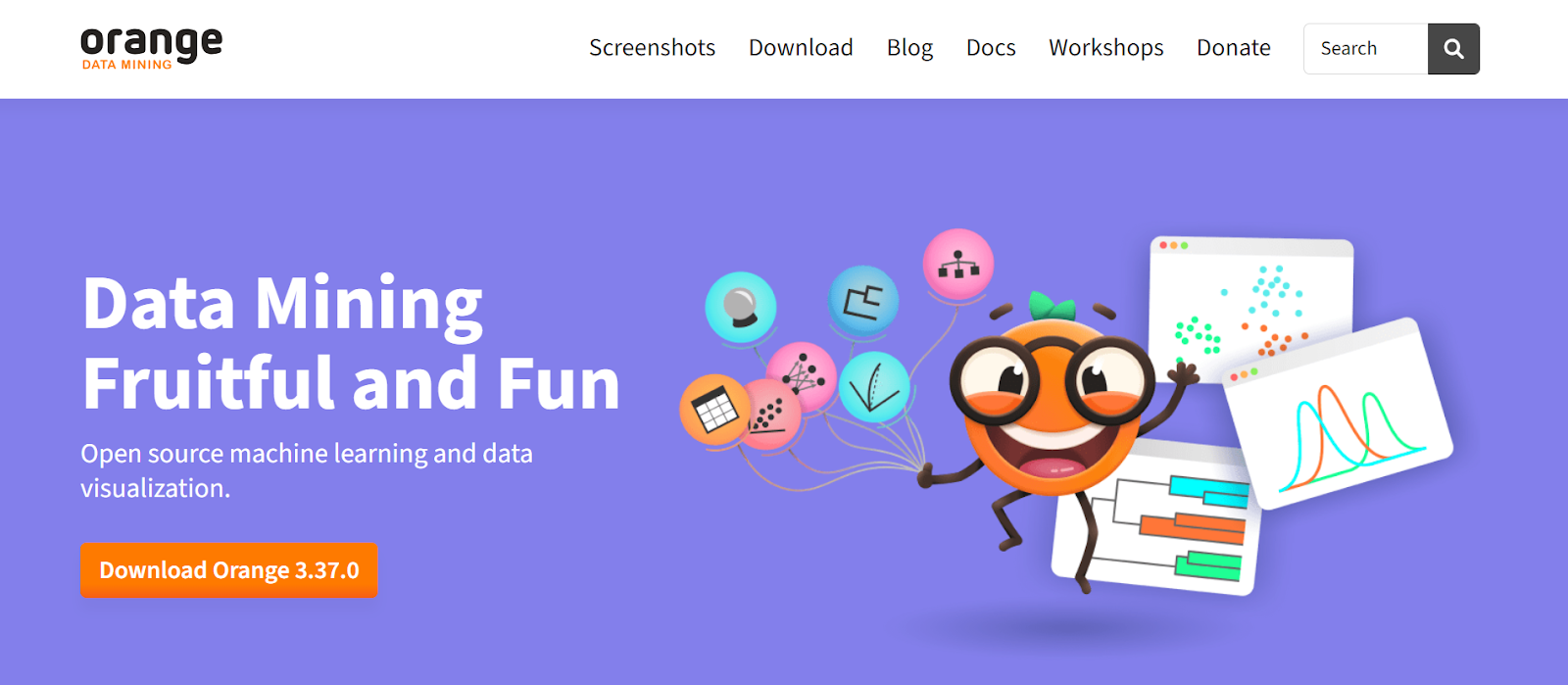
Orange is another data mining and machine learning project that provides a computational platform and Python API. It can be used for a variety of financial analysis tasks. Including fraud detection and Risk assessment
Key Points:
- It creates models visually without writing code.
- It creates clear visualisations to analyse data.
- Access to algorithm libraries for modelling.
Pros:
- Easy to learn and use even for beginners.
- It has a wide range of data visualisation and analysis tools.
- It is tightly integrated with Python for more advanced customisation.
- Active community and many online resources
Cons:
- This may be limiting for complex models or large data sets.
- May not have as many features as other tools
- There may be limitations for highly customised models.
- May not be appropriate for companies with highly structured data.
More Info: Orange Data Mining
6. Dataiku DSS
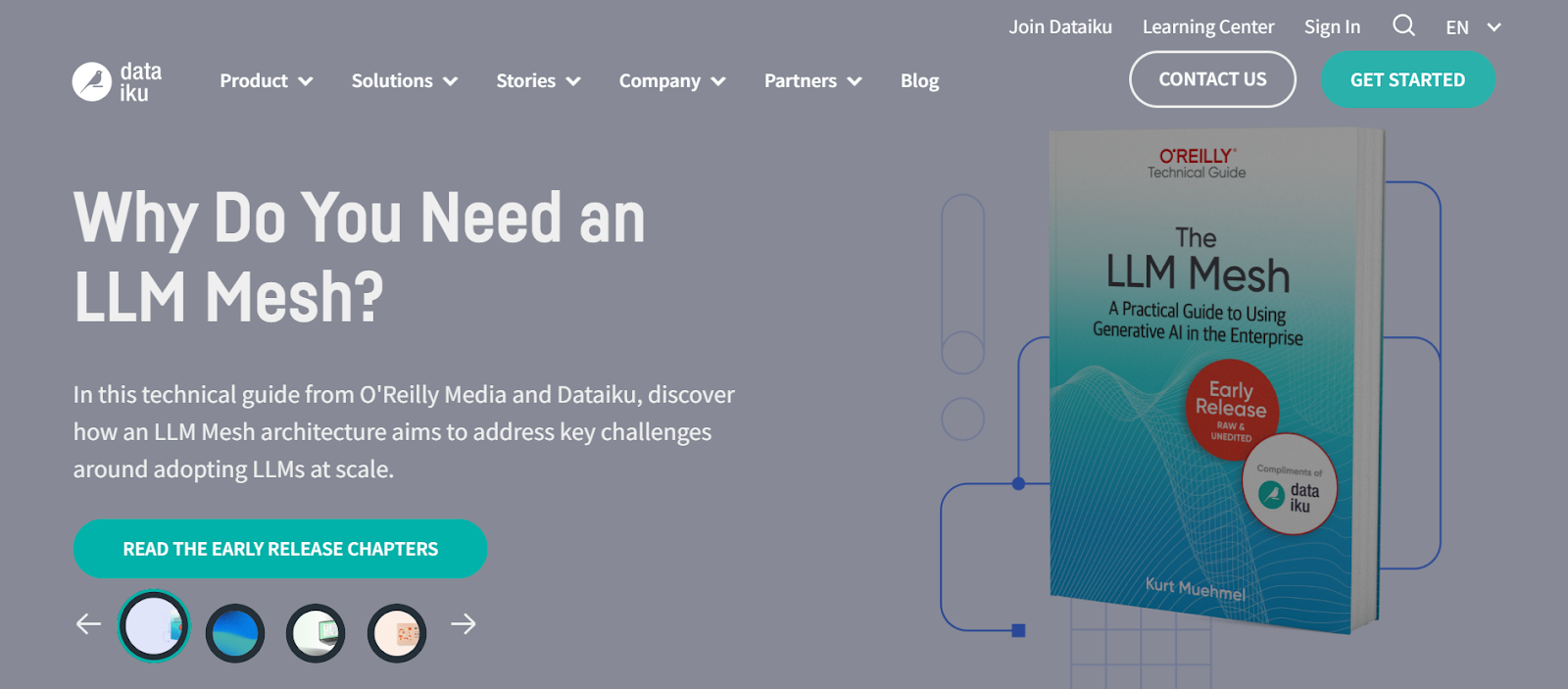
Dataiku DSS is a collaborative database that provides a unified framework for data processing, exploration, and modelling. It can be used for a variety of financial analysis tasks. Including fraud detection risk assessment and notifying customers.
Key Points:
- It works with data canters and analyst teams.
- Cleaning organizing and data analysis
- Access to algorithm libraries for modeling.
- Deploying the sample to workstations
Pros:
- It’s very powerful and scalable for large data center projects.
- Collaborative space for team projects
- There are a variety of data science tools available.
- Mix with other media very well
Cons:
- It can be expensive compared to other open source tools.
- It can be a steep learning curve for beginners.
- There may be limitations for highly customised models.
- May not be appropriate for companies with highly structured data.
More Info: Dataiku
7. Weka
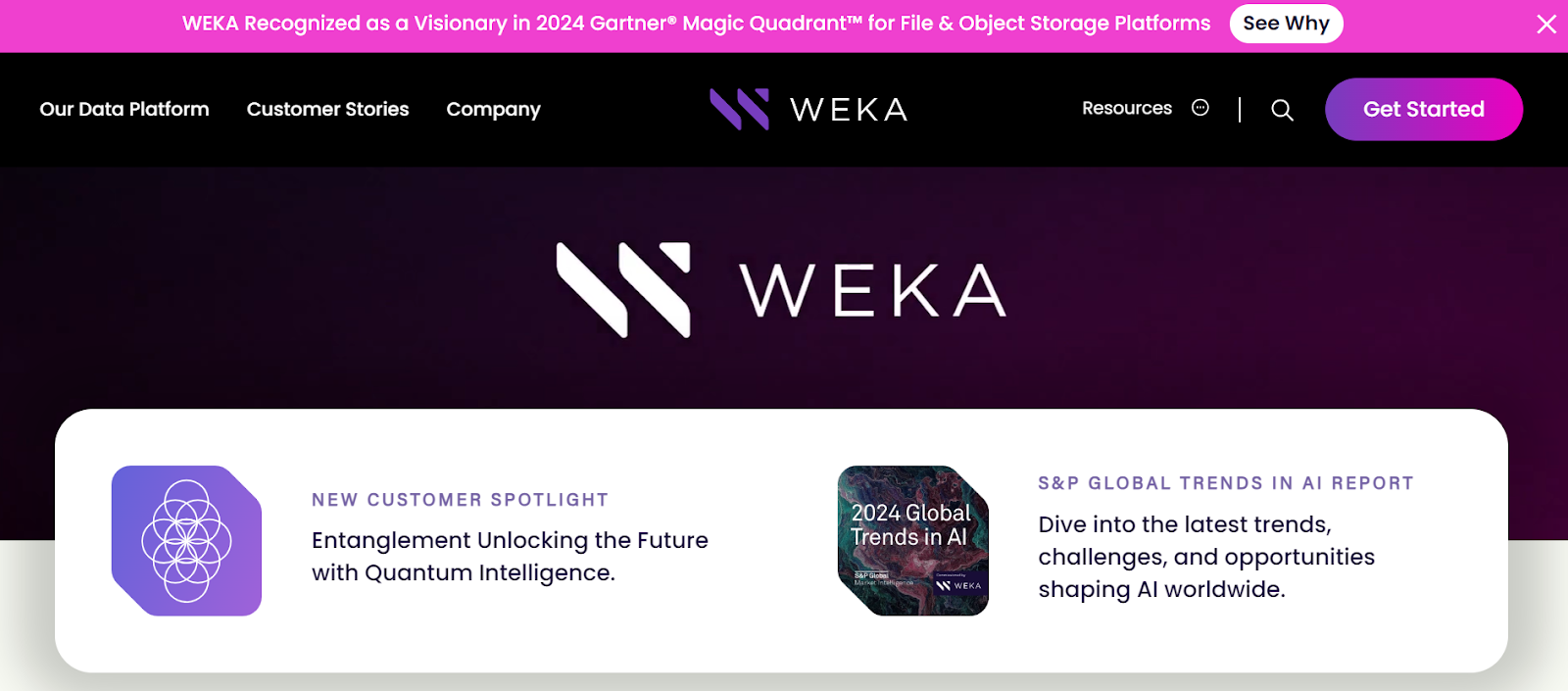
Weka is a popular open source machine learning software that provides a set of algorithms for data mining applications. Can be used for a variety of financial analysis tasks.
Key Points:
- You can have multiple algorithms for searching the database.
- Create models visually without writing code.
- free and customisable
- Data cleaning and preprocessing.
Pros:
- Very flexible and adjustable
- It can be used for a variety of data mining applications.
- A great and enthusiastic community for support and resources.
- It integrates well with other Java-based applications.
Cons:
- It can be a steep learning curve for beginners.
- It may not be as easy as other tools.
- There may be limitations for highly customised models.
- May not be appropriate for companies with highly structured data.
More Info: Weka
8. H2O.ai
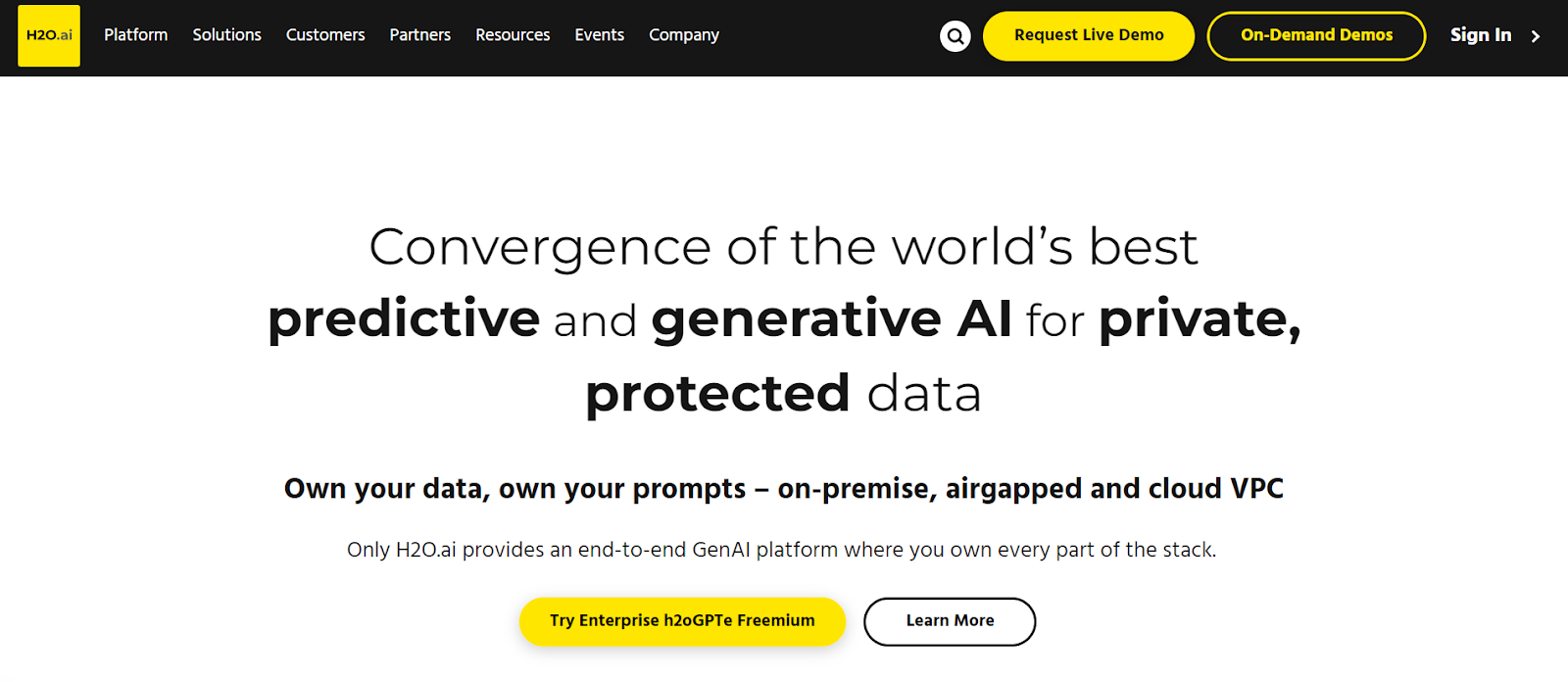
H2O.ai is a scalable machine learning platform. It provides a variety of tools to create and deploy predictive models. Can be used for a variety of financial analysis tasks.
Key Points:
- Managing Big Data and Complex
- You can access the site from a web browser.
- Connect to Python for advanced customisation.
- Automating model creation and optimisation processes.
Pros:
- It is highly scalable and works well in large-scale machine learning applications. Users often use it online.
- It is closely integrated with Python and other programming languages.
- Features automatic machine learning for rapid model development
Cons:
- Can be expensive compared to other open source tools
- Programming knowledge may be required to successfully adapt.
- There may be limitations for highly customised models.
- May not be appropriate for companies with highly structured data.
More Info: H2O.ai
9. TensorFlow
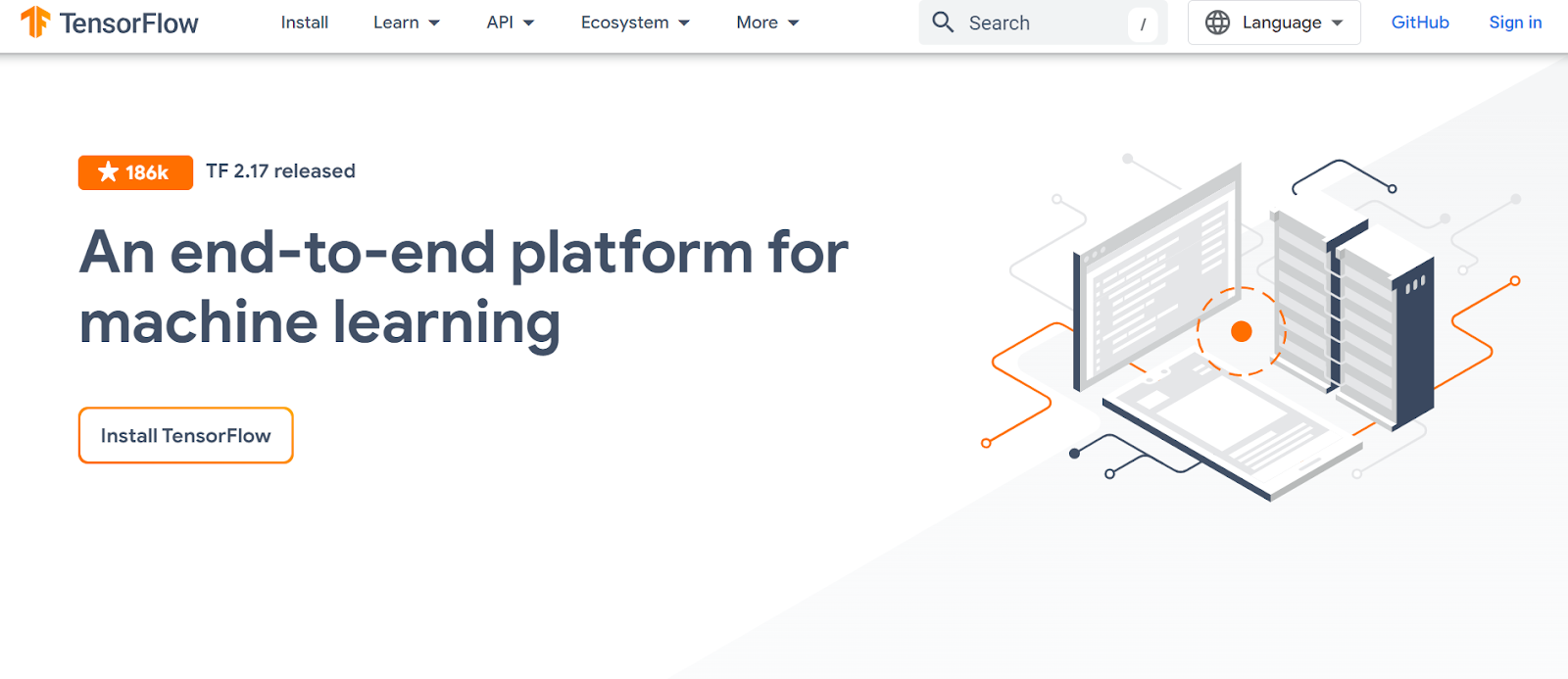
TensorFlow is an open source machine learning platform that can be used in a variety of financial analysis applications. Including fraud detection Risk assessment and notifying customers
Key Points:
- Building and Training Customisable Machine Learning Models
- free and customisable
- Manage large data sets and complex models.
- Connecting to other data sources.
Pros:
- Very flexible and adjustable
- It can be used in many Machine Learning applications.
- A great and enthusiastic community for support and resources.
- Mix with other media very well
Cons:
- It requires strong programming skills and knowledge of machine learning concepts.
- It can be a steep learning curve for beginners.
- Additional technical expertise may be required for more complex models.
- May not be appropriate for companies with highly structured data.
Credit: TensorFlow
10. PyTorch
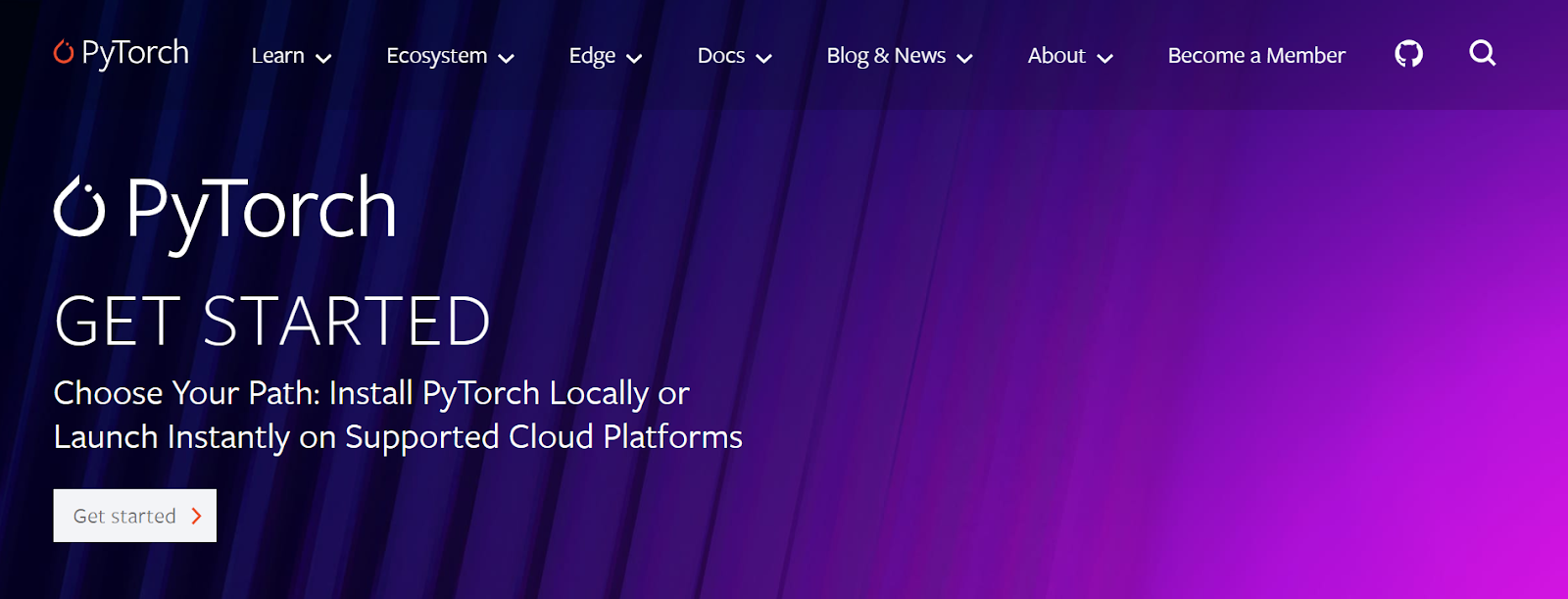
PyTorch is another open source machine learning platform that can be used for a variety of financial analysis tasks. Which includes fraud detection, Risk assessment and notifying customers
Key Points:
- creating and upgrading models
- free and customisable
- Manage large data sets and complex models.
- Connecting to other data sources
Pros:
- Very flexible and adjustable
- It can be used in many Machine Learning applications.
- A great and enthusiastic community for support and resources.
- Mix with other media very well
Cons:
- It requires strong programming skills and knowledge of machine learning concepts.
- It can be a steep learning curve for beginners.
- Additional technical expertise may be required for more complex models.
- May not be appropriate for companies with highly structured data.
More Info: PyTorch
Wrap UP: Boost Your Finance with These 10 AI Tools
Combining AI with financial analysis can provide immediate, reliable insights. While automating complex tasks, these top 10 AI tools to transform financial analysis can help simplify data analysis.
Clarifying trends and reducing human error in investment decisions. Using these tools, businesses and investment managers will gain a competitive advantage and make smart and data-driven decisions.